Response to letter

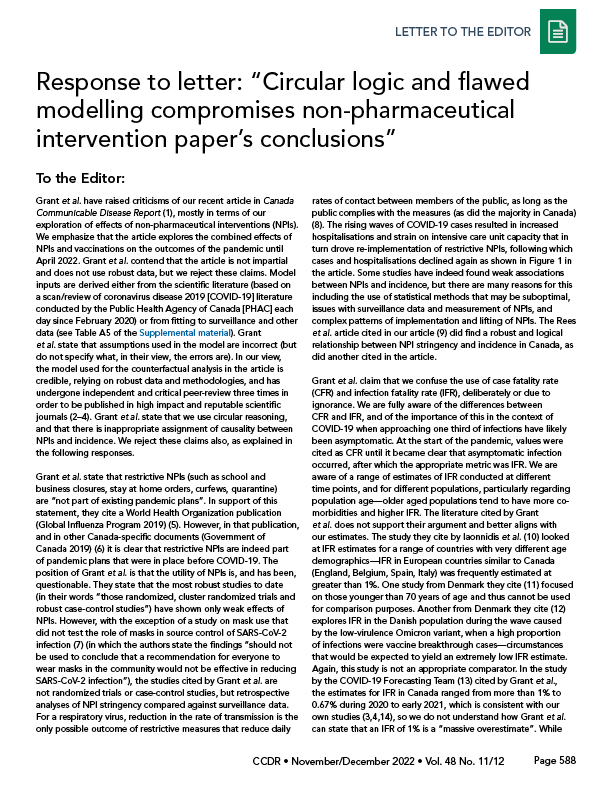
Published by: The Public Health Agency of Canada
Issue: Volume 48-11/12, November/December 2022: Antimicrobial Use and Stewardship
Date published: November/December 2022
ISSN: 1481-8531
Submit a manuscript
About CCDR
Browse
Volume 48-11/12, November/December 2022: Antimicrobial Use and Stewardship
Letter to the Editor
Response to letter: “Circular logic and flawed modelling compromises non-pharmaceutical intervention paper’s conclusions”
To the Editor:
Grant et al. have raised criticisms of our recent article in Canada Communicable Disease Report Footnote 1, mostly in terms of our exploration of effects of non-pharmaceutical interventions (NPIs). We emphasize that the article explores the combined effects of NPIs and vaccinations on the outcomes of the pandemic until April 2022. Grant et al. contend that the article is not impartial and does not use robust data, but we reject these claims. Model inputs are derived either from the scientific literature (based on a scan/review of coronavirus disease 2019 [COVID-19] literature conducted by the Public Health Agency of Canada [PHAC] each day since February 2020) or from fitting to surveillance and other data (see Table A5 of the Supplemental material). Grant et al. state that assumptions used in the model are incorrect (but do not specify what, in their view, the errors are). In our view, the model used for the counterfactual analysis in the article is credible, relying on robust data and methodologies, and has undergone independent and critical peer-review three times in order to be published in high impact and reputable scientific journals Footnote 2 Footnote 3 Footnote 4. Grant et al. state that we use circular reasoning, and that there is inappropriate assignment of causality between NPIs and incidence. We reject these claims also, as explained in the following responses.
Grant et al. state that restrictive NPIs (such as school and business closures, stay at home orders, curfews, quarantine) are “not part of existing pandemic plans”. In support of this statement, they cite a World Health Organization publication (Global Influenza Program 2019) Footnote 5. However, in that publication, and in other Canada-specific documents (Government of Canada 2019) Footnote 6 it is clear that restrictive NPIs are indeed part of pandemic plans that were in place before COVID-19. The position of Grant et al. is that the utility of NPIs is, and has been, questionable. They state that the most robust studies to date (in their words “those randomized, cluster randomized trials and robust case-control studies”) have shown only weak effects of NPIs. However, with the exception of a study on mask use that did not test the role of masks in source control of SARS-CoV-2 infection Footnote 7 (in which the authors state the findings “should not be used to conclude that a recommendation for everyone to wear masks in the community would not be effective in reducing SARS-CoV-2 infection”), the studies cited by Grant et al. are not randomized trials or case-control studies, but retrospective analyses of NPI stringency compared against surveillance data. For a respiratory virus, reduction in the rate of transmission is the only possible outcome of restrictive measures that reduce daily rates of contact between members of the public, as long as the public complies with the measures (as did the majority in Canada) Footnote 8. The rising waves of COVID-19 cases resulted in increased hospitalisations and strain on intensive care unit capacity that in turn drove re-implementation of restrictive NPIs, following which cases and hospitalisations declined again as shown in Figure 1 in the article. Some studies have indeed found weak associations between NPIs and incidence, but there are many reasons for this including the use of statistical methods that may be suboptimal, issues with surveillance data and measurement of NPIs, and complex patterns of implementation and lifting of NPIs. The Rees et al. article cited in our article Footnote 9 did find a robust and logical relationship between NPI stringency and incidence in Canada, as did another cited in the article.
Grant et al. claim that we confuse the use of case fatality rate (CFR) and infection fatality rate (IFR), deliberately or due to ignorance. We are fully aware of the differences between CFR and IFR, and of the importance of this in the context of COVID-19 when approaching one third of infections have likely been asymptomatic. At the start of the pandemic, values were cited as CFR until it became clear that asymptomatic infection occurred, after which the appropriate metric was IFR. We are aware of a range of estimates of IFR conducted at different time points, and for different populations, particularly regarding population age—older aged populations tend to have more co-morbidities and higher IFR. The literature cited by Grant et al. does not support their argument and better aligns with our estimates. The study they cite by Iaonnidis et al. Footnote 10 looked at IFR estimates for a range of countries with very different age demographics—IFR in European countries similar to Canada (England, Belgium, Spain, Italy) was frequently estimated at greater than 1%. One study from Denmark they cite Footnote 11 focused on those younger than 70 years of age and thus cannot be used for comparison purposes. Another from Denmark they cite Footnote 12 explores IFR in the Danish population during the wave caused by the low-virulence Omicron variant, when a high proportion of infections were vaccine breakthrough cases—circumstances that would be expected to yield an extremely low IFR estimate. Again, this study is not an appropriate comparator. In the study by the COVID-19 Forecasting Team Footnote 13 cited by Grant et al., the estimates for IFR in Canada ranged from more than 1% to 0.67% during 2020 to early 2021, which is consistent with our own studies Footnote 3 Footnote 4 Footnote 14, so we do not understand how Grant et al. can state that an IFR of 1% is a “massive overestimate”. While IFR likely decreased during the first year of the pandemic as therapies improved (as indicated by the study of the COVID-19 Forecasting Team) Footnote 13, IFR subsequently increased due to the emergence of the more virulent Alpha and Delta variants, and the combined impact of these factors are accounted for in counterfactual modelling. It is clear in the methods, and by viewing Table 3, that the model fully accounts for asymptomatic infections, and the model outputs of symptomatic (likely to be “cases”) and asymptomatic infections (unlikely to be “cases”) are explicitly stated. Grant et al. appear to assume that estimates of IFR cited in the article come from a simple calculation of data presented on reported cases and deaths in Table 1 of the article, but it is explicit in the table that the number of cases recorded in surveillance underestimate the true number of infections, and citations are provided for IFR estimates.
Grant et al. state that we relied on “flawed and discredited mathematical models” citing an article by Ioannidis et al. Footnote 15. This article reviewed outcomes of model-based forecasting, which is not the type of model used in our study. It is true that models parameterized with incorrect parameter values, or fit to data that are unsound, will likely produce inaccurate results. However, forecasts by good models often do not come to pass, particularly if, based on the forecasts, policies change to increase control of the epidemic. The model used for the counterfactual analysis is an agent-based computational model that simulates actions and interactions of individuals and is particularly suited for studying the effectiveness of different scenarios of interventions (in contrast to forecasts) that are highly dependent on community and population dynamics, such as the effectiveness of NPIs and vaccines.
Grant et al. claim that the model “presumes efficacy of NPIs to prove that NPIs have efficacy” and that “this circular reasoning alone should have disqualified this paper at the stage of peer review”. In the paper, we do not “presume” efficacy of NPIs. We do indeed cite articles that support the efficacy of NPIs, and we visually compare incidence and NPI stringency (in Figure 1), but we then model impacts of implementation and lifting of NPIs in Canada. In the model, NPIs have an impact on contacts between agents (i.e. members of the public) or transmission probability when contacts occur, which is informed by estimates from the scientific literature (a scan and review of COVID-19 literature is conducted by PHAC each day), from Canadian data sources (e.g. hospital occupancy, vaccine uptake, open-source mobility data) or from fitting to surveillance data. For example, restrictive closures were modelled on the reduction in mobility from open-access, population-level data associated with changes in the stringency index, and associated reductions of contact rates were based on surveys of the Canadian public that have been conducted at multiple time points during the pandemic. The model inputs (all described in the Supplemental material) therefore reflected changes in NPIs at various times of the pandemic according to what actually occurred, while the model outputs assessed the efficacy of these NPIs on the epidemic. There is not, therefore, a circular argument associated with us selecting unrealistically high effectiveness values for NPIs in the modelling because simple effectiveness values for NPIs are not model inputs.
Grant et al. suggest our worst-case upper bound estimate of 800,000 deaths in the counterfactual analysis is unreasonable. They estimate that this would mean an IFR of 3% and a death rate fourteen times higher than that seen in Sweden. We emphasize that this worst-case counterfactual estimate obtained in the model does not include vaccination and accounts for waning of post-infection immunity acquired in wave 1 (according to current estimates of waning of immunity against infection and severe outcomes) allowing a large wave of reinfections associated with the more virulent Delta wave to occur (see Figure 4). Overall IFR in this counterfactual scenario remains 1% as can be estimated from the model output data in Table 3. It is not correct to compare this counterfactual estimate with observed data from Sweden where both NPIs and vaccinations were implemented.
Grant et al. appear to assume that Figure 1 in the article, which compares the timelines of variations in stringency of NPIs and incidence of COVID-19, is used to attribute causality of incidence to NPI stringency. However, Figure 1 is merely a pictorial description of the timeline of the epidemic and implementation and release of NPIs. It is a simplification as, of course, there were inter-provincial variations in the timing of implementation and release of different NPIs. There is no attempt to infer causality from this diagram; causality is inferred from more detail statistical analyses cited in the article Footnote 8 Footnote 16.
Grant et al. claim that we did not consider other explanations, including the lower death rates in British Columbia compared to Québec when stringency was higher in the latter province and that death rates are affected by factors such as age structure, obesity rate, population density and economic disparity. In our experience, stringency and mortality rates varied amongst provinces and territories according to a number of factors including the number of cases detected initially during the pandemic, the intrinsic within-province or territory characteristics of transmission, healthcare capacity etc. This article looked at Canada as a whole rather than dissecting regional variations, but in the article we point out the value of future jurisdiction-by-jurisdiction analyses. Some interprovincial differences in fatality rates are associated with epidemics in long-term care, that were more severe in some provinces than others, and it should be noted that some inter-provincial disparities are due to differences in completeness of reporting of cases and deaths. Reporting of deaths in Québec was likely more complete than other provinces Footnote 17. The epidemics that occurred in long-term care are not considered in, nor do they inflate, outputs from counterfactual modelling because the model represents the baseline number of infections, hospitalisations and deaths excluding outbreaks such as those seen in long-term care facilities, hospitals and other localized outbreaks (see the Supplemental material). The counterfactual modelling is therefore a conservative estimate of the efficacy of NPIs and vaccination in Canada. Further, the mortality rate that was used in the counterfactual modelling was derived from national surveillance data of the first 200,000 cases reported in Canada; this inherently takes into account some of the complexities that Grant et al. has pointed out including age structure, population density, socioeconomic disparity and comorbidities such as obesity that varies across Canada.
Grant et al. criticize the article for choosing inappropriate comparator nations (“two isolated islands [New Zealand and Australia] and a country without functional land borders [South Korea]”) and state that “the authors…..conveniently forget that these countries have subsequently had massive outbreaks”. The zero-COVID approach to managing COVID-19 was presented in our article as an alternative that was adopted by certain countries or jurisdictions. It was made clear that this approach was only possible under certain circumstances as stated by Grant et al. Those that did adopt this approach had fewer deaths per capita than countries that did not, up to early 2022 when the Omicron variant emerged, and NPIs in these countries were lifted. After NPIs were lifted, and transmission of COVID-19 was unrestricted, there was an expected significant increase in infections and deaths in these countries, as occurred in many countries including Canada with the lifting of NPIs. It is made clear in the article that “as the Omicron variant emerged, most of these countries experienced major outbreaks…” once NPIs were lifted. Hospitalisations and deaths occurred in zero-COVID countries during the Omicron waves because, despite high levels of vaccine uptake, many people remained unvaccinated and, of course, while the vaccines are very effective against severe outcomes, they are not 100% effective. Even so, to date the rates of deaths in Australia and New Zealand (circa 57 and 39 per 100,000 population at the time of writing) are substantially lower than in Canada (at the time of writing 118 per 100,000) and in the Unites States (at the time of writing 311 per 100,000).
Grant et al. criticise the authors for not considering unintended consequences of NPIs. It is made clear in the article that exploring COVID-19 cases and deaths is our starting point for exploring counterfactuals, but the article shows the potentially catastrophic impact of COVID-19 in Canada had public health measures and vaccination not been implemented as they were. As mentioned in the article, future studies are needed to explore the full range of consequences of COVID-19, long-COVID and unintended consequences of NPIs, which are beyond the scope of this paper. One way of exploring the full impact is by excess deaths. While there is a perception that deaths due to unintended consequences of NPIs may be substantial, there is not much evidence, with some exceptions such as the impact on overdose deaths in British Columbia. In the zero-COVID countries, deaths were generally lower than in years prior to the pandemic, possibly due to NPIs reducing transmission of a range of other infectious diseases Footnote 18 Footnote 19. When analyses have found significant excess deaths over and above reported deaths, these have been mostly attributable to under-reporting of COVID-19 deaths, rather than to deaths due to unintended consequences of NPIs Footnote 18. It should also be recognized that delays in diagnosis and treatment for non-COVID-19 illnesses such as cancer were likely due to hospital capacity being overwhelmed with COVID-19 patients. It has been argued that late re-implementation of restrictions to control transmission contributed to non-COVID-19 deaths due to deferral of routine diagnostic and surgical procedures Footnote 20 Footnote 21 Footnote 22.
Grant et al. consider that the author team, as members of PHAC, would actually have a competing interest in producing a favourable evaluation of the management of the epidemic in Canada because of our responsibility for decision-making. This perception is not correct. During a pandemic, PHAC officials, and the Chief Public Health Officer Footnote 23, have a key role in providing advice—evidence-based recommendations and best practice guidance, and all the authors were involved in contributing to the development and communication of this scientific information. In addition, the PHAC has key roles in acting as a central national focus for liaison with domestic and international partners and for facilitating public health action. With the exception of measures at our international borders, the decisions on implementation of NPIs and administration of vaccines have always been the responsibility of provincial and territorial governments in consultation with their own public health advisors. While PHAC developed guidance and recommendations to facilitate responses by provinces and territories, the overall pattern of changes in NPIs during the pandemic, as described in Figure 1, comes from decisions made at the provincial and territorial level. In this article, we therefore comment on what occurred as informed observers of the implementation and lifting of NPIs—not as architects. We emphasize that this article aims to describe what could have happened with lower levels of use of NPIs and of vaccine uptake. It shows that outcomes in terms of COVID-19 cases, hospitalizations and deaths may have been far worse than actually occurred by comparing against counterfactuals in a modelling study and comparing against outcomes in other countries. It does not explore whether or not management of the pandemic in Canada was optimal, and we were explicit that further study of that is needed.
References
- Footnote 1
-
Ogden NH, Turgeon P, Fazil A, Clark J, Gabriele-Rivet V, Tam T, Ng V. Counterfactuals of effects of vaccination and public health measures on COVID-19 cases in Canada: what could have happened? Can Commun Dis Rep 2022;48(7/8):292–302. https://doi.org/10.14745/ccdr.v48i78a01
- Footnote 2
-
Ng V, Fazil A, Waddell LA, Bancej C, Turgeon P, Otten A, Atchessi N, Ogden NH. Projected effects of nonpharmaceutical public health interventions to prevent resurgence of SARS-CoV-2 transmission in Canada. CMAJ 2020;192(37):E1053–64. https://doi.org/10.1503/cmaj.200990
- Footnote 3
-
Ng V, Fazil A, Waddell LA, Turgeon P, Otten A, Ogden NH. Modelling the impact of shutdowns on resurging SARS-CoV-2 transmission in Canada. R Soc Open Sci 2021;8(5):210233. https://doi.org/10.1098/rsos.210233
- Footnote 4
-
Gabriele-Rivet V, Spence KL, Ogden NH, Fazil A, Turgeon P, Otten A, Waddell LA, Ng V. Modelling the impact of age-stratified public health measures on SARS-CoV-2 transmission in Canada. R Soc Open Sci 2021;8(11):210834. https://doi.org/10.1098/rsos.210834
- Footnote 5
-
World Health Organization. Global Influenza Program. Non-pharmaceutical public health measures for mitigating the risk and impact of epidemic and pandemic influenza. Genva (CH): WHO; 2019. https://www.who.int/publications/i/item/non-pharmaceutical-public-health-measuresfor-mitigating-the-risk-and-impact-of-epidemic-and-pandemic-influenza
- Footnote 6
-
Public Health Agency of Canada. Public health measures: Canadian Pandemic Influenza Preparedness: Planning Guidance for the Health Sector. Ottawa, ON: PHAC; 2019. https://www.canada.ca/en/public-health/services/flu-influenza/canadian-pandemic-influenza-preparedness-planning-guidance-health-sector/public-health-measures.html
- Footnote 7
-
Bundgaard H, Bundgaard JS, Raaschou-Pedersen DET, von Buchwald C, Todsen T, Norsk JB, Pries-Heje MM, Vissing CR, Nielsen PB, Winsløw UC, Fogh K, Hasselbalch R, Kristensen JH, Ringgaard A, Porsborg Andersen M, Goecke NB, Trebbien R, Skovgaard K, Benfield T, Ullum H, Torp-Pedersen C, Iversen K. Effectiveness of Adding a Mask Recommendation to Other Public Health Measures to Prevent SARS-CoV-2 Infection in Danish Mask Wearers : A Randomized Controlled Trial. Ann Intern Med 2021;174(3):335–343. https://doi.org/10.7326/M20-6817
- Footnote 8
-
Brankston G, Merkley E, Loewen PJ, Avery BP, Carson CA, Dougherty BP, Fisman DN, Tuite AR, Poljak Z, Greer AL. Pandemic fatigue or enduring precautionary behaviours? Canadians’ long-term response to COVID-19 public health measures. Prev Med Rep 2022;30:101993. https://doi.org/10.1016/j.pmedr.2022.101993
- Footnote 9
-
Rees EE, Avery BP, Carabin H, Carson CA, Champredon D, de Montigny S, Dougherty B, Nasri BR, Ogden NH. Effectiveness of non-pharmaceutical interventions to reduce SARS-CoV-2 transmission in Canada and their association with COVID-19 hospitalization rates. Can Commun Dis Rep 2022;48(10):438–48. https://doi.org/10.14745/ccdr.v48i10a04
- Footnote 10
-
Ioannidis JP. Infection fatality rate of COVID-19 inferred from seroprevalence data. Bull World Health Organ 2021;99(1):19–33F. https://doi.org/10.2471/BLT.20.265892
- Footnote 11
-
Kaspersen KA, Hindhede L, Boldsen JK, Mikkelsen S, Vestergaard LS, Berthelsen AN, Moustsen-Helms IR, Holm DK, Nilsson AC, Sækmose SG, Sørensen E, Harritshøj LH, Aagaard B, Hjalgrim H, Lillevang ST, Jørgensen CS, Krause TG, Ullum H, Pedersen OB, Ostrowski SR, Erikstrup C. Estimation of SARS-CoV-2 infection fatality rate by age and comorbidity status using antibody screening of blood donors during the COVID-19 epidemic in Denmark. J Infect Dis 2022;225(2):219–28. https://doi.org/10.1093/infdis/jiab566
- Footnote 12
-
Erikstrup C, Laksafoss AD, Gladov J, Kaspersen KA, Mikkelsen S, Hindhede L, Boldsen JK, Jørgensen SW, Ethelberg S, Holm DK, Bruun MT, Nissen J, Schwinn M, Brodersen T, Mikkelsen C, Sækmose SG, Sørensen E, Harritshøj LH, Aagaard B, Dinh KM, Busch MP, Jørgensen CS, Krause TG, Ullum H, Ostrowski SR, Espenhain L, Pedersen OBV. Seroprevalence and infection fatality rate of the SARS-CoV-2 Omicron variant in Denmark: A nationwide serosurveillance study. Lancet Reg Health Eur 2022;21:100479. https://doi.org/10.1016/j.lanepe.2022.100479
- Footnote 13
-
COVID-19 Forecasting Team. Variation in the COVID-19 infection-fatality ratio by age, time, and geography during the pre-vaccine era: a systematic analysis. Lancet 2022;399(10334):1469–88. https://doi.org/10.1016/S0140-6736(21)02867-1
- Footnote 14
-
Dougherty BP, Smith BA, Carson CA, Ogden NH. Exploring the percentage of COVID-19 cases reported in the community in Canada and associated case fatality ratios. Infect Dis Model 2021;6:123–32. https://doi.org/10.1016/j.idm.2020.11.008
- Footnote 15
-
Ioannidis JP, Cripps S, Tanner MA. Forecasting for COVID-19 has failed. Int J Forecast 2022;38(2):423–38. https://doi.org/10.1016/j.ijforecast.2020.08.004
- Footnote 16
-
Haug N, Geyrhofer L, Londei A, Dervic E, Desvars-Larrive A, Loreto V, Pinior B, Thurner S, Klimek P. Ranking the effectiveness of worldwide COVID-19 government interventions. Nat Hum Behav 2020;4(12):1303–12. https://doi.org/10.1038/s41562-020-01009-0
- Footnote 17
-
Royal Society of Canada. Excess all-cause mortality during the COVID-19 epidemic in Canada. Ottawa, ON: RSC; 2021. https://rsc-src.ca/en/covid-19-policy-briefing/excess-all-cause-mortality-during-covid-19-epidemic-in-canada
- Footnote 18
-
COVID-19 Excess Mortality Collaborators. Estimating excess mortality due to the COVID-19 pandemic: a systematic analysis of COVID-19-related mortality, 2020-21. Lancet 2022;399(10334):1513–36. https://doi.org/10.1016/S0140-6736(21)02796-3
- Footnote 19
-
Meyerowitz-Katz G, Bhatt S, Ratmann O, Brauner JM, Flaxman S, Mishra S, Sharma M, Mindermann S, Bradley V, Vollmer M, Merone L, Yamey G. Is the cure really worse than the disease? The health impacts of lockdowns during COVID-19. BMJ Glob Health 2021;6(8):e006653. https://doi.org/10.1136/bmjgh-2021-006653
- Footnote 20
-
Duong D. Doctors warn of late diagnoses as cancer screening backlog grows. CMAJ 2021;193(22):E811–2. https://doi.org/10.1503/cmaj.1095944
- Footnote 21
-
Malagón T, Yong JH, Tope P, Miller WH Jr, Franco EL; McGill Task Force on the Impact of COVID-19 on Cancer Control and Care. Predicted long-term impact of COVID-19 pandemic-related care delays on cancer mortality in Canada. Int J Cancer 2022;150(8):1244–54. https://doi.org/10.1002/ijc.33884
- Footnote 22
-
Ontario COVID-19 Science Advisory Table. Critical care capacity during the COVID-19 pandemic. Science Table; 2021. https://covid19-sciencetable.ca/sciencebrief/critical-care-capacity-during-the-covid-19-pandemic/
- Footnote 23
-
Public Health Agency of Canada. The Role of the Chief Public Health Officer. Ottawa, ON: PHAC; 2016. https://www.canada.ca/en/public-health/corporate/organizational-structure/canada-chief-public-health-officer/role-chief-public-health-officer.html
Page details
- Date modified: